Research Topics
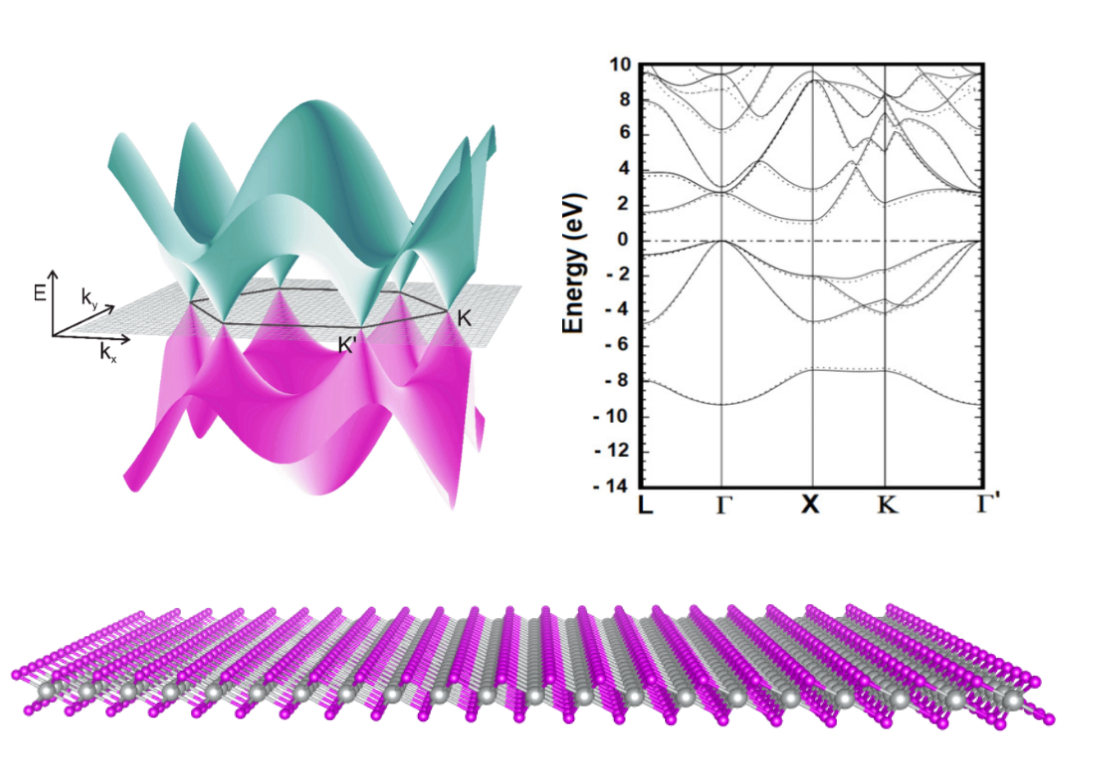
Quantum Mechanical Models to Unveil the Secrets of Novel Materials
The Condensed Matter Theory Group stands at the forefront of unraveling the complexities of novel materials through the lens of quantum mechanics. This sophisticated approach lies at the heart of our understanding of the underlying principles that dictate the behavior of matter in its condensed phases. By employing quantum mechanical calculations, our research team delves deep into the microscopic world, revealing the interactions and phenomena that are crucial in the development of advanced technological applications. At the core of our research lies the commitment to a profound understanding of the fundamental mechanisms governing the physics of novel materials. Quantum mechanics, with its ability to describe the behavior of particles at the smallest scales, provides an indispensable framework. We utilize a variety of quantum mechanical models, including ab initio methods and density functional theory, to simulate and predict the behavior of materials with astonishing accuracy. These theoretical models allow us to explore the electronic, magnetic, and optical properties of materials, offering insights into their behavior at an atomic level.
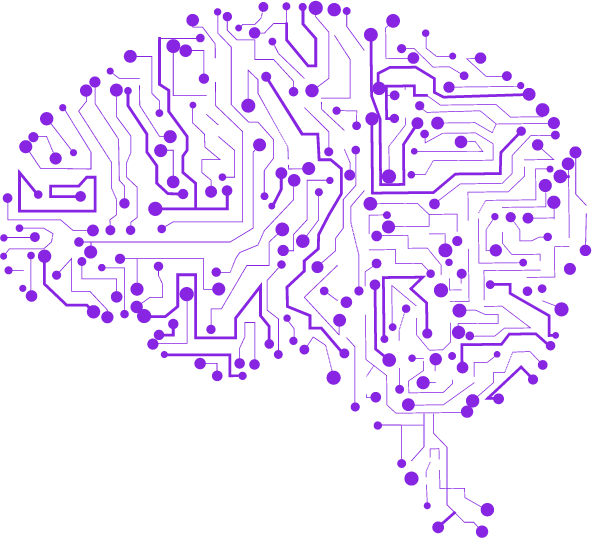
Machine Learning: A New Frontier in Condensed Matter Theory
In the evolving landscape of condensed matter physics, the integration of machine learning models marks a transformative shift in our approach to studying and understanding complex materials. The Condensed Matter Theory Group is at the vanguard of this exciting intersection, leveraging the power of machine learning to uncover new insights and propel material science into a new era. By processing large datasets derived from experimental and computational sources, these models can identify patterns and correlations that are imperceptible to conventional analysis methods. In our research, we employ a variety of machine learning techniques, from neural networks to decision trees, to decipher the complex relationships between the atomic structure of materials and their physical properties. This innovative approach enables us to predict the behavior of materials with unprecedented speed and accuracy.
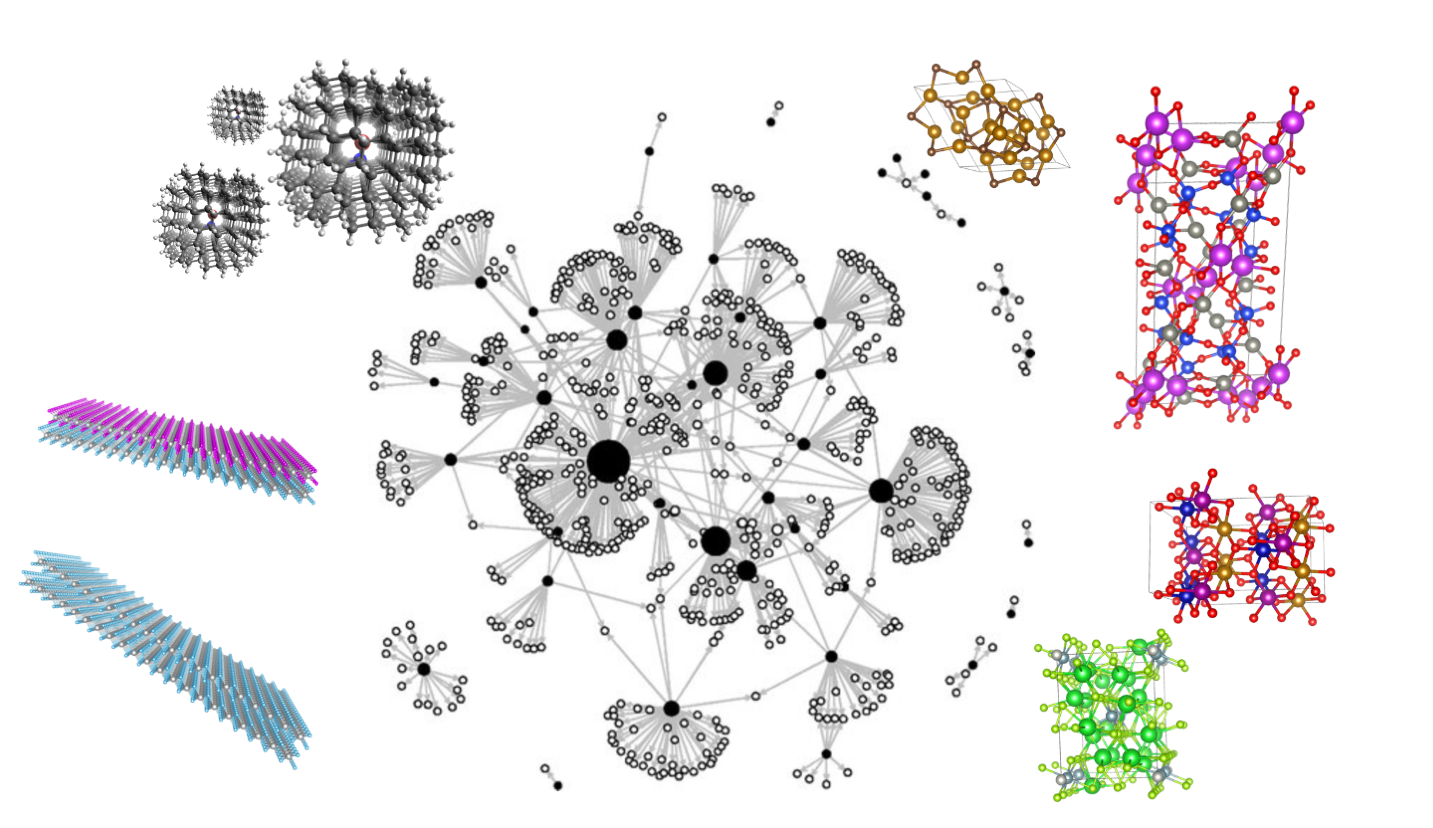
Materials Discovery: Accelerating the Pace of Material Innovation
One of the most significant impacts of machine learning in condensed matter theory is the acceleration of material discovery and design. Traditional methods of material synthesis and characterization are often time-consuming and resource-intensive. Machine learning models, with their ability to quickly analyze vast datasets, offer a more efficient route to exploring the material landscape. We harness these models to predict new materials with desired properties, significantly reducing the trial-and-error process in laboratories. This not only speeds up the discovery of novel materials but also paves the way for designing materials withe specific applications in mind, such as energy storage, photonics, and nanotechnology.